Elements of Probability Theory and Statistical Data Analysis
Contents
1. Elements of Probability Theory and Statistical Data Analysis¶
1.1. Domains and probabilities¶
Consider the following simple example, namely the tossing of two dice, resulting in the following possible values
These values are called the domain. To this domain we have the corresponding probabilities
The numbers in the domain are the outcomes of the physical process of tossing say two dice. We cannot tell beforehand whether the outcome is 3 or 5 or any other number in this domain. This defines the randomness of the outcome, or unexpectedness or any other synonimous word which encompasses the uncertitude of the final outcome.
The only thing we can tell beforehand
is that say the outcome 2 has a certain probability.
If our favorite hobby is to spend an hour every evening throwing dice and
registering the sequence of outcomes, we will note that the numbers in the above domain
appear in a random order. After 11 throws the results may look like
Random variables are characterized by a domain which contains all possible values that the random value may take. This domain has a corresponding probability distribution function(PDF).
1.1.1. Stochastic variables and the main concepts, the discrete case¶
There are two main concepts associated with a stochastic variable. The domain is the set \(\mathbb D = \{x\}\) of all accessible values the variable can assume, so that \(X \in \mathbb D\). An example of a discrete domain is the set of six different numbers that we may get by throwing of a dice, \(x\in\{1,\,2,\,3,\,4,\,5,\,6\}\).
The probability distribution function (PDF) is a function \(p(x)\) on the domain which, in the discrete case, gives us the probability or relative frequency with which these values of \(X\) occur
In the continuous case, the PDF does not directly depict the actual probability. Instead we define the probability for the stochastic variable to assume any value on an infinitesimal interval around \(x\) to be \(p(x)dx\). The continuous function \(p(x)\) then gives us the density of the probability rather than the probability itself. The probability for a stochastic variable to assume any value on a non-infinitesimal interval \([a,\,b]\) is then just the integral
Qualitatively speaking, a stochastic variable represents the values of numbers chosen as if by chance from some specified PDF so that the selection of a large set of these numbers reproduces this PDF.
Of interest to us is the cumulative probability distribution function (CDF), \(P(x)\), which is just the probability for a stochastic variable \(X\) to assume any value less than \(x\)
The relation between a CDF and its corresponding PDF is then
1.1.2. Properties of PDFs¶
There are two properties that all PDFs must satisfy. The first one is positivity (assuming that the PDF is normalized)
Naturally, it would be nonsensical for any of the values of the domain to occur with a probability greater than \(1\) or less than \(0\). Also, the PDF must be normalized. That is, all the probabilities must add up to unity. The probability of “anything” to happen is always unity. For both discrete and continuous PDFs, this condition is
The first one is the most basic PDF; namely the uniform distribution
For \(a=0\) and \(b=1\) we have
The latter distribution is used to generate random numbers. For other PDFs, one needs normally a mapping from this distribution to say for example the exponential distribution.
The second one is the Gaussian Distribution
with mean value \(\mu\) and standard deviation \(\sigma\). If \(\mu=0\) and \(\sigma=1\), it is normally called the standard normal distribution
The following simple Python code plots the above distribution for different values of \(\mu\) and \(\sigma\).
%matplotlib inline
import numpy as np
from math import acos, exp, sqrt
from matplotlib import pyplot as plt
from matplotlib import rc, rcParams
import matplotlib.units as units
import matplotlib.ticker as ticker
rc('text',usetex=True)
rc('font',**{'family':'serif','serif':['Gaussian distribution']})
font = {'family' : 'serif',
'color' : 'darkred',
'weight' : 'normal',
'size' : 16,
}
pi = acos(-1.0)
mu0 = 0.0
sigma0 = 1.0
mu1= 1.0
sigma1 = 2.0
mu2 = 2.0
sigma2 = 4.0
x = np.linspace(-20.0, 20.0)
v0 = np.exp(-(x*x-2*x*mu0+mu0*mu0)/(2*sigma0*sigma0))/sqrt(2*pi*sigma0*sigma0)
v1 = np.exp(-(x*x-2*x*mu1+mu1*mu1)/(2*sigma1*sigma1))/sqrt(2*pi*sigma1*sigma1)
v2 = np.exp(-(x*x-2*x*mu2+mu2*mu2)/(2*sigma2*sigma2))/sqrt(2*pi*sigma2*sigma2)
plt.plot(x, v0, 'b-', x, v1, 'r-', x, v2, 'g-')
plt.title(r'{\bf Gaussian distributions}', fontsize=20)
plt.text(-19, 0.3, r'Parameters: $\mu = 0$, $\sigma = 1$', fontdict=font)
plt.text(-19, 0.18, r'Parameters: $\mu = 1$, $\sigma = 2$', fontdict=font)
plt.text(-19, 0.08, r'Parameters: $\mu = 2$, $\sigma = 4$', fontdict=font)
plt.xlabel(r'$x$',fontsize=20)
plt.ylabel(r'$p(x)$ [MeV]',fontsize=20)
# Tweak spacing to prevent clipping of ylabel
plt.subplots_adjust(left=0.15)
plt.savefig('gaussian.pdf', format='pdf')
plt.show()
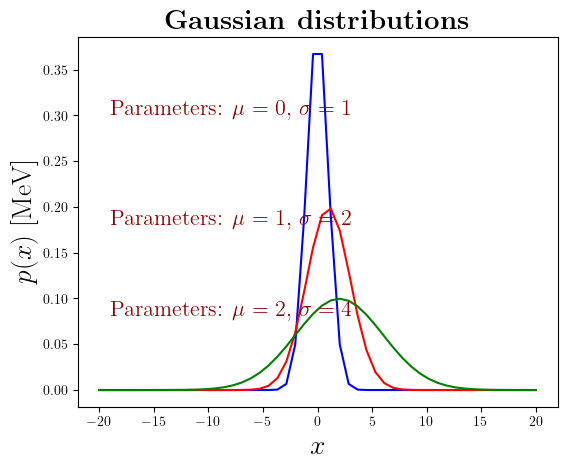
Another important distribution in science is the exponential distribution
1.1.3. Expectation values¶
Let \(h(x)\) be an arbitrary continuous function on the domain of the stochastic variable \(X\) whose PDF is \(p(x)\). We define the expectation value of \(h\) with respect to \(p\) as follows
Whenever the PDF is known implicitly, like in this case, we will drop
the index \(X\) for clarity.
A particularly useful class of special expectation values are the
moments. The \(n\)-th moment of the PDF \(p\) is defined as
follows
The zero-th moment \(\langle 1\rangle\) is just the normalization condition of \(p\). The first moment, \(\langle x\rangle\), is called the mean of \(p\) and often denoted by the letter \(\mu\)
for a continuous distribution and
for a discrete distribution. Qualitatively it represents the centroid or the average value of the PDF and is therefore simply called the expectation value of \(p(x)\).
A special version of the moments is the set of central moments, the n-th central moment defined as
The zero-th and first central moments are both trivial, equal \(1\) and \(0\), respectively. But the second central moment, known as the variance of \(p\), is of particular interest. For the stochastic variable \(X\), the variance is denoted as \(\sigma^2_X\) or \(\mathrm{Var}(X)\)
The square root of the variance, \(\sigma =\sqrt{\langle (x-\langle x\rangle)^2\rangle}\) is called the standard deviation of \(p\). It is the RMS (root-mean-square) value of the deviation of the PDF from its mean value, interpreted qualitatively as the “spread” of \(p\) around its mean.
1.1.4. Probability Distribution Functions¶
The following table collects properties of probability distribution functions. In our notation we reserve the label \(p(x)\) for the probability of a certain event, while \(P(x)\) is the cumulative probability.
Discrete PDF | Continuous PDF | |
---|---|---|
Domain | $\left\{x_1, x_2, x_3, \dots, x_N\right\}$ | $[a,b]$ |
Probability | $p(x_i)$ | $p(x)dx$ |
Cumulative | $P_i=\sum_{l=1}^ip(x_l)$ | $P(x)=\int_a^xp(t)dt$ |
Positivity | $0 \le p(x_i) \le 1$ | $p(x) \ge 0$ |
Positivity | $0 \le P_i \le 1$ | $0 \le P(x) \le 1$ |
Monotonic | $P_i \ge P_j$ if $x_i \ge x_j$ | $P(x_i) \ge P(x_j)$ if $x_i \ge x_j$ |
Normalization | $P_N=1$ | $P(b)=1$ |
With a PDF we can compute expectation values of selected quantities such as
if we have a discrete PDF or
in the case of a continuous PDF. We have already defined the mean value \(\mu\) and the variance \(\sigma^2\).
There are at least three PDFs which one may encounter. These are the
Uniform distribution
yielding probabilities different from zero in the interval \([a,b]\).
The exponential distribution
yielding probabilities different from zero in the interval \([0,\infty)\) and with mean value
with variance
Finally, we have the so-called univariate normal distribution, or just the normal distribution
with probabilities different from zero in the interval \((-\infty,\infty)\). The integral \(\int_{-\infty}^{\infty}\exp{\left(-(x^2\right)}dx\) appears in many calculations, its value is \(\sqrt{\pi}\), a result we will need when we compute the mean value and the variance. The mean value is
which becomes with a suitable change of variables
Similarly, the variance becomes
and inserting the mean value and performing a variable change we obtain
and performing a final integration by parts we obtain the well-known result \(\sigma^2=b^2\). It is useful to introduce the standard normal distribution as well, defined by \(\mu=a=0\), viz. a distribution centered around zero and with a variance \(\sigma^2=1\), leading to
The exponential and uniform distributions have simple cumulative functions, whereas the normal distribution does not, being proportional to the so-called error function \(erf(x)\), given by
which is difficult to evaluate in a quick way.
Some other PDFs which one encounters often in the natural sciences are the binomial distribution
where \(y\) is the probability for a specific event, such as the tossing of a coin or moving left or right in case of a random walker. Note that \(x\) is a discrete stochastic variable.
The sequence of binomial trials is characterized by the following definitions
Every experiment is thought to consist of \(N\) independent trials.
In every independent trial one registers if a specific situation happens or not, such as the jump to the left or right of a random walker.
The probability for every outcome in a single trial has the same value, for example the outcome of tossing (either heads or tails) a coin is always \(1/2\).
In order to compute the mean and variance we need to recall Newton’s binomial formula
which can be used to show that
the PDF is normalized to one. The mean value is
resulting in
which we rewrite as
The variance is slightly trickier to get. It reads \(\sigma^2=ny(1-y)\).
Another important distribution with discrete stochastic variables \(x\) is
the Poisson model, which resembles the exponential distribution and reads
In this case both the mean value and the variance are easier to calculate,
and the variance is \(\sigma^2=\lambda\).
An example of applications of the Poisson distribution could be the counting of the number of \(\alpha\)-particles emitted from a radioactive source in a given time interval. In the limit of \(n\rightarrow \infty\) and for small probabilities \(y\), the binomial distribution approaches the Poisson distribution. Setting \(\lambda = ny\), with \(y\) the probability for an event in the binomial distribution we can show that
1.1.5. Meet the covariance!¶
An important quantity in a statistical analysis is the so-called covariance.
Consider the set \(\{X_i\}\) of \(n\) stochastic variables (not necessarily uncorrelated) with the multivariate PDF \(P(x_1,\dots,x_n)\). The covariance of two of the stochastic variables, \(X_i\) and \(X_j\), is defined as follows
with
If we consider the above covariance as a matrix
then the diagonal elements are just the familiar variances, \(C_{ii} = \mathrm{Cov}(X_i,\,X_i) = \mathrm{Var}(X_i)\). It turns out that all the off-diagonal elements are zero if the stochastic variables are uncorrelated.
# Importing various packages
from math import exp, sqrt
from random import random, seed
import numpy as np
import matplotlib.pyplot as plt
def covariance(x, y, n):
sum = 0.0
mean_x = np.mean(x)
mean_y = np.mean(y)
for i in range(0, n):
sum += (x[(i)]-mean_x)*(y[i]-mean_y)
return sum/n
n = 10
x=np.random.normal(size=n)
y = 4+3*x+np.random.normal(size=n)
covxy = covariance(x,y,n)
print(covxy)
z = np.vstack((x, y))
c = np.cov(z.T)
print(c)
3.56625717949593
[[ 1.06428266 0.38523213 1.79328214 2.08565179 2.50663068 6.8647244
3.38978208 3.14317326 1.71034931 3.18370342]
[ 0.38523213 0.13944021 0.64910378 0.7549311 0.90731036 2.48478389
1.22697947 1.13771593 0.61908508 1.15238639]
[ 1.79328214 0.64910378 3.02162289 3.51425636 4.22359229 11.56684041
5.71167406 5.29614612 2.88188373 5.36443813]
[ 2.08565179 0.7549311 3.51425636 4.08720685 4.91219011 13.45265242
6.64288286 6.15960888 3.35173468 6.23903496]
[ 2.50663068 0.90731036 4.22359229 4.91219011 5.90369232 16.16800633
7.98371718 7.40289664 4.02826638 7.49835449]
[ 6.8647244 2.48478389 11.56684041 13.45265242 16.16800633 44.27812536
21.86441684 20.27376652 11.03191576 20.53518988]
[ 3.38978208 1.22697947 5.71167406 6.64288286 7.98371718 21.86441684
10.79658906 10.01113029 5.44752974 10.14022043]
[ 3.14317326 1.13771593 5.29614612 6.15960888 7.40289664 20.27376652
10.01113029 9.28281416 5.05121846 9.4025129 ]
[ 1.71034931 0.61908508 2.88188373 3.35173468 4.02826638 11.03191576
5.44752974 5.05121846 2.748607 5.11635222]
[ 3.18370342 1.15238639 5.36443813 6.23903496 7.49835449 20.53518988
10.14022043 9.4025129 5.11635222 9.52375513]]
Consider the stochastic variables \(X_i\) and \(X_j\), (\(i\neq j\)). We have
If \(X_i\) and \(X_j\) are independent (assuming \(i \neq j\)), we have that
leading to
Now that we have constructed an idealized mathematical framework, let us try to apply it to empirical observations. Examples of relevant physical phenomena may be spontaneous decays of nuclei, or a purely mathematical set of numbers produced by some deterministic mechanism. It is the latter we will deal with, using so-called pseudo-random number generators. In general our observations will contain only a limited set of observables. We remind the reader that a stochastic process is a process that produces sequentially a chain of values
We will call these values our measurements and the entire set as our measured sample. The action of measuring all the elements of a sample we will call a stochastic experiment (since, operationally, they are often associated with results of empirical observation of some physical or mathematical phenomena; precisely an experiment). We assume that these values are distributed according to some PDF \(p_X^{\phantom X}(x)\), where \(X\) is just the formal symbol for the stochastic variable whose PDF is \(p_X^{\phantom X}(x)\). Instead of trying to determine the full distribution \(p\) we are often only interested in finding the few lowest moments, like the mean \(\mu_X^{\phantom X}\) and the variance \(\sigma_X^{\phantom X}\).
In practical situations however, a sample is always of finite size. Let that size be \(n\). The expectation value of a sample \(\alpha\), the sample mean, is then defined as follows
The sample variance is:
with its square root being the standard deviation of the sample.
You can think of the above observables as a set of quantities which define a given experiment. This experiment is then repeated several times, say \(m\) times. The total average is then
where the last sums end at \(m\) and \(n\). The total variance is
which we rewrite as
We define also the sample variance \(\sigma^2\) of all \(mn\) individual experiments as
These quantities, being known experimental values or the results from our calculations, may differ, in some cases significantly, from the similarly named exact values for the mean value \(\mu_X\), the variance \(\mathrm{Var}(X)\) and the covariance \(\mathrm{Cov}(X,Y)\).
1.1.6. Numerical experiments and the covariance, central limit theorem¶
The central limit theorem states that the PDF \(\tilde{p}(z)\) of the average of \(m\) random values corresponding to a PDF \(p(x)\) is a normal distribution whose mean is the mean value of the PDF \(p(x)\) and whose variance is the variance of the PDF \(p(x)\) divided by \(m\), the number of values used to compute \(z\).
The central limit theorem leads then to the well-known expression for the standard deviation, given by
In many cases the above estimate for the standard deviation, in particular if correlations are strong, may be too simplistic. We need therefore a more precise defintion of the error and the variance in our results.
Our estimate of the true average \(\mu_{X}\) is the sample mean \(\langle X_m \rangle\)
We can then use Eq. (7)
and rewrite it as
where the first term is the sample variance of all \(mn\) experiments divided by \(n\) and the last term is nothing but the covariance which arises when \(k\ne l\).
Our estimate of the true average \(\mu_{X}\) is the sample mean \(\langle X_m \rangle\)
If the observables are uncorrelated, then the covariance is zero and we obtain a total variance which agrees with the central limit theorem. Correlations may often be present in our data set, resulting in a non-zero covariance. The first term is normally called the uncorrelated contribution. Computationally the uncorrelated first term is much easier to treat efficiently than the second. We just accumulate separately the values \(x^2\) and \(x\) for every measurement \(x\) we receive. The correlation term, though, has to be calculated at the end of the experiment since we need all the measurements to calculate the cross terms. Therefore, all measurements have to be stored throughout the experiment.
Let us analyze the problem by splitting up the correlation term into partial sums of the form
The correlation term of the total variance can now be rewritten in terms of \(f_d\)
The value of \(f_d\) reflects the correlation between measurements separated by the distance \(d\) in the samples. Notice that for \(d=0\), \(f\) is just the sample variance, \(\sigma^2\). If we divide \(f_d\) by \(\sigma^2\), we arrive at the so called autocorrelation function
which gives us a useful measure of the correlation pair correlation starting always at \(1\) for \(d=0\).
The sample variance of the \(mn\) experiments can now be written in terms of the autocorrelation function
and we see that \(\sigma_m\) can be expressed in terms of the uncorrelated sample variance times a correction factor \(\tau\) which accounts for the correlation between measurements. We call this correction factor the autocorrelation time
For a correlation free experiment, \(\tau\) equals 1.
From the point of view of Eq. (10) we can interpret a sequential correlation as an effective reduction of the number of measurements by a factor \(\tau\). The effective number of measurements becomes
To neglect the autocorrelation time \(\tau\) will always cause our simple uncorrelated estimate of \(\sigma_m^2\approx \sigma^2/n\) to be less than the true sample error. The estimate of the error will be too “good”. On the other hand, the calculation of the full autocorrelation time poses an efficiency problem if the set of measurements is very large. The solution to this problem is given by more practically oriented methods like the blocking technique.
# Importing various packages
from math import exp, sqrt
from random import random, seed
import numpy as np
import matplotlib.pyplot as plt
# Sample covariance, note the factor 1/(n-1)
def covariance(x, y, n):
sum = 0.0
mean_x = np.mean(x)
mean_y = np.mean(y)
for i in range(0, n):
sum += (x[(i)]-mean_x)*(y[i]-mean_y)
return sum/(n-1.)
n = 100
x = np.random.normal(size=n)
print(np.mean(x))
y = 4+3*x+np.random.normal(size=n)
print(np.mean(y))
z = x**3+np.random.normal(size=n)
print(np.mean(z))
covxx = covariance(x,x,n)
covyy = covariance(y,y,n)
covzz = covariance(z,z,n)
covxy = covariance(x,y,n)
covxz = covariance(x,z,n)
covyz = covariance(y,z,n)
print(covxx,covyy, covzz)
print(covxy,covxz, covyz)
w = np.vstack((x, y, z))
#print(w)
c = np.cov(w)
print(c)
#eigen = np.zeros(n)
Eigvals, Eigvecs = np.linalg.eig(c)
print(Eigvals)
0.15035891139512705
4.404353162658163
0.26135632456837465
0.8498535115108263 9.637848274275777 7.478236340866993
2.683498103515284 1.8820155508745924 5.929759221040619
[[0.84985351 2.6834981 1.88201555]
[2.6834981 9.63784827 5.92975922]
[1.88201555 5.92975922 7.47823634]]
[15.32272016 0.08352309 2.55969487]
1.1.7. Random Numbers¶
Uniform deviates are just random numbers that lie within a specified range (typically 0 to 1), with any one number in the range just as likely as any other. They are, in other words, what you probably think random numbers are. However, we want to distinguish uniform deviates from other sorts of random numbers, for example numbers drawn from a normal (Gaussian) distribution of specified mean and standard deviation. These other sorts of deviates are almost always generated by performing appropriate operations on one or more uniform deviates, as we will see in subsequent sections. So, a reliable source of random uniform deviates, the subject of this section, is an essential building block for any sort of stochastic modeling or Monte Carlo computer work.
A disclaimer is however appropriate. It should be fairly obvious that something as deterministic as a computer cannot generate purely random numbers.
Numbers generated by any of the standard algorithms are in reality pseudo random numbers, hopefully abiding to the following criteria:
they produce a uniform distribution in the interval [0,1].
correlations between random numbers are negligible
the period before the same sequence of random numbers is repeated is as large as possible and finally
the algorithm should be fast.
The most common random number generators are based on so-called Linear congruential relations of the type
which yield a number in the interval [0,1] through
The number \(M\) is called the period and it should be as large as possible and \(N_0\) is the starting value, or seed. The function \(\mathrm{MOD}\) means the remainder, that is if we were to evaluate \((13)\mathrm{MOD}(9)\), the outcome is the remainder of the division \(13/9\), namely \(4\).
The problem with such generators is that their outputs are periodic; they will start to repeat themselves with a period that is at most \(M\). If however the parameters \(a\) and \(c\) are badly chosen, the period may be even shorter.
Consider the following example
with a seed \(N_0=2\). This generator produces the sequence \(4,1,3,0,2,4,1,3,0,2,...\dots\), i.e., a sequence with period \(5\). However, increasing \(M\) may not guarantee a larger period as the following example shows
which still, with \(N_0=2\), results in \(11,38,11,38,11,38,\dots\), a period of just \(2\).
Typical periods for the random generators provided in the program library are of the order of \(\sim 10^9\) or larger. Other random number generators which have become increasingly popular are so-called shift-register generators. In these generators each successive number depends on many preceding values (rather than the last values as in the linear congruential generator). For example, you could make a shift register generator whose \(l\)th number is the sum of the \(l-i\)th and \(l-j\)th values with modulo \(M\),
Such a generator again produces a sequence of pseudorandom numbers but this time with a period much larger than \(M\). It is also possible to construct more elaborate algorithms by including more than two past terms in the sum of each iteration. One example is the generator of Marsaglia and Zaman which consists of two congruential relations
followed by
which according to the authors has a period larger than \(2^{94}\).
Instead of using modular addition, we could use the bitwise exclusive-OR (\(\oplus\)) operation so that
where the bitwise action of \(\oplus\) means that if \(N_{l-i}=N_{l-j}\) the result is \(0\) whereas if \(N_{l-i}\ne N_{l-j}\) the result is \(1\). As an example, consider the case where \(N_{l-i}=6\) and \(N_{l-j}=11\). The first one has a bit representation (using 4 bits only) which reads \(0110\) whereas the second number is \(1011\). Employing the \(\oplus\) operator yields \(1101\), or \(2^3+2^2+2^0=13\).
In Fortran90, the bitwise \(\oplus\) operation is coded through the intrinsic function \(\mathrm{IEOR}(m,n)\) where \(m\) and \(n\) are the input numbers, while in \(C\) it is given by \(m\wedge n\).
We show here how the linear congruential algorithm can be implemented, namely
However, since \(a\) and \(N_{i-1}\) are integers and their multiplication could become greater than the standard 32 bit integer, there is a trick via Schrage’s algorithm which approximates the multiplication of large integers through the factorization
where we have defined
and
where the brackets denote integer division. In the code below the numbers \(q\) and \(r\) are chosen so that \(r < q\).
To see how this works we note first that
since we can add or subtract any integer multiple of \(M\) from \(aN_{i-1}\). The last term \([N_{i-1}/q]M\mathrm{MOD}(M)\) is zero since the integer division \([N_{i-1}/q]\) just yields a constant which is multiplied with \(M\).
We can now rewrite Eq. (14) as
which results
yielding
The term \([N_{i-1}/q]r\) is always smaller or equal \(N_{i-1}(r/q)\) and with \(r < q\) we obtain always a number smaller than \(N_{i-1}\), which is smaller than \(M\). And since the number \(N_{i-1}\mathrm{MOD} (q)\) is between zero and \(q-1\) then \(a(N_{i-1}\mathrm{MOD} (q))< aq\). Combined with our definition of \(q=[M/a]\) ensures that this term is also smaller than \(M\) meaning that both terms fit into a 32-bit signed integer. None of these two terms can be negative, but their difference could. The algorithm below adds \(M\) if their difference is negative. Note that the program uses the bitwise \(\oplus\) operator to generate the starting point for each generation of a random number. The period of \(ran0\) is \(\sim 2.1\times 10^{9}\). A special feature of this algorithm is that is should never be called with the initial seed set to \(0\).
As mentioned previously, the underlying PDF for the generation of random numbers is the uniform distribution, meaning that the probability for finding a number \(x\) in the interval [0,1] is \(p(x)=1\).
A random number generator should produce numbers which are uniformly distributed in this interval. The table shows the distribution of \(N=10000\) random numbers generated by the functions in the program library. We note in this table that the number of points in the various intervals \(0.0-0.1\), \(0.1-0.2\) etc are fairly close to \(1000\), with some minor deviations.
Two additional measures are the standard deviation \(\sigma\) and the mean \(\mu=\langle x\rangle\).
For the uniform distribution, the mean value \(\mu\) is then
while the standard deviation is
The various random number generators produce results which agree rather well with these limiting values.
$x$-bin | ran0 | ran1 | ran2 | ran3 |
---|---|---|---|---|
0.0-0.1 | 1013 | 991 | 938 | 1047 |
0.1-0.2 | 1002 | 1009 | 1040 | 1030 |
0.2-0.3 | 989 | 999 | 1030 | 993 |
0.3-0.4 | 939 | 960 | 1023 | 937 |
0.4-0.5 | 1038 | 1001 | 1002 | 992 |
0.5-0.6 | 1037 | 1047 | 1009 | 1009 |
0.6-0.7 | 1005 | 989 | 1003 | 989 |
0.7-0.8 | 986 | 962 | 985 | 954 |
0.8-0.9 | 1000 | 1027 | 1009 | 1023 |
0.9-1.0 | 991 | 1015 | 961 | 1026 |
$\mu$ | 0.4997 | 0.5018 | 0.4992 | 0.4990 |
$\sigma$ | 0.2882 | 0.2892 | 0.2861 | 0.2915 |
The following simple Python code plots the distribution of the produced random numbers using the linear congruential RNG employed by Python. The trend displayed in the previous table is seen rather clearly.
#!/usr/bin/env python
import numpy as np
import matplotlib.mlab as mlab
import matplotlib.pyplot as plt
import random
# initialize the rng with a seed
random.seed()
counts = 10000
values = np.zeros(counts)
for i in range (1, counts, 1):
values[i] = random.random()
# the histogram of the data
n, bins, patches = plt.hist(values, 10, facecolor='green')
plt.xlabel('$x$')
plt.ylabel('Number of counts')
plt.title(r'Test of uniform distribution')
plt.axis([0, 1, 0, 1100])
plt.grid(True)
plt.show()
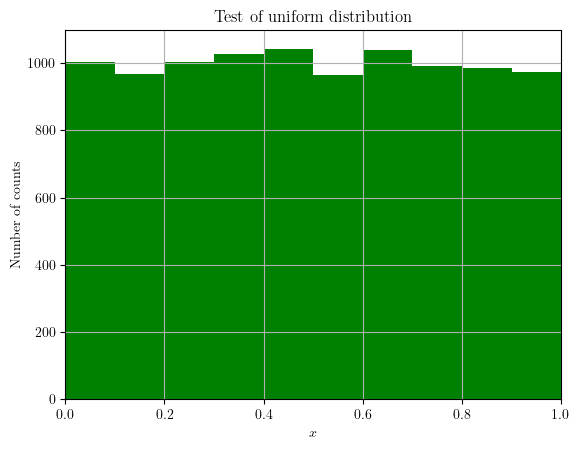
Since our random numbers, which are typically generated via a linear congruential algorithm,
are never fully independent, we can then define
an important test which measures the degree of correlation, namely the so-called
auto-correlation function defined previously, see again Eq. (9).
We rewrite it here as
with \(C_0=1\). Recall that \(\sigma^2=\langle x_i^2\rangle-\langle x_i\rangle^2\) and that
The non-vanishing of \(C_k\) for \(k\ne 0\) means that the random numbers are not independent. The independence of the random numbers is crucial in the evaluation of other expectation values. If they are not independent, our assumption for approximating \(\sigma_N\) is no longer valid.
1.1.8. Autocorrelation function¶
This program computes the autocorrelation function as discussed in the equation on the previous slide for random numbers generated with the normal distribution \(N(0,1)\).
# Importing various packages
from math import exp, sqrt
from random import random, seed
import numpy as np
import matplotlib.pyplot as plt
def autocovariance(x, n, k, mean_x):
sum = 0.0
for i in range(0, n-k):
sum += (x[(i+k)]-mean_x)*(x[i]-mean_x)
return sum/n
n = 1000
x=np.random.normal(size=n)
autocor = np.zeros(n)
figaxis = np.zeros(n)
mean_x=np.mean(x)
var_x = np.var(x)
print(mean_x, var_x)
for i in range (0, n):
figaxis[i] = i
autocor[i]=(autocovariance(x, n, i, mean_x))/var_x
plt.plot(figaxis, autocor, "r-")
plt.axis([0,n,-0.1, 1.0])
plt.xlabel(r'$i$')
plt.ylabel(r'$\gamma_i$')
plt.title(r'Autocorrelation function')
plt.show()
-0.030334875296061884 0.9620141923630161
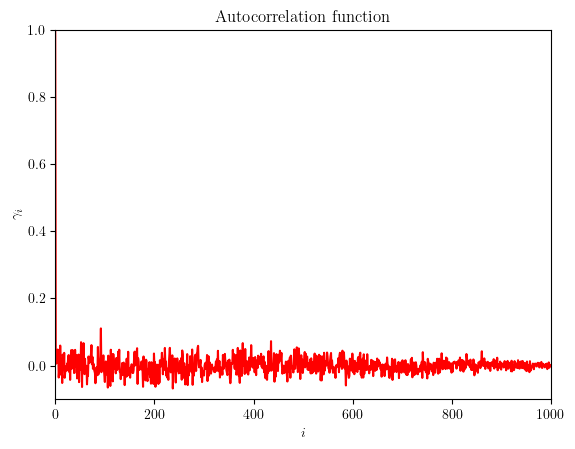
As can be seen from the plot, the first point gives back the variance and a value of one. For the remaining values we notice that there are still non-zero values for the auto-correlation function.